Interpreting and Visualizing Principal Components Using Cornerstone
Dimensionality reduction is an important step in data analysis, especially when dealing with big datasets. One popular method for this is Principal Component Analysis (PCA) which is a core Cornerstone. With some simple tricks, the software makes it possible to interpret and visualize the Principal Component (PC) loadings in a compact and clear way. To show this, we have used the solar panel production records from Cornerstone’s sample data and conducted a PCA. The original data set contains around 7,300 observations and 114 potential predictors. The PCA reduces these factors to 20 PCs which explains more than 96% of the total variation. Further, we have created a Tile Map Graph on a reshaped loadings table. To make the graph even more interactive, we added a reference line which resorts to the original variables by loading size. As a result, we obtain a powerful tool to explore the loadings for each PC. This graph can be recreated for each PCA, independent of the number of PCs or predictors one wishes to examine. Interpreting the PCs helps explain the relation between predictors and responses in further analyses
/New%20Whitepaper%20Thumbnail/Whitepaper_Interpreting%20and%20Visualizing.webp)
Download Here
Explore More Pages
Discover valuable resources—from our latest news, events, and webinars to white papers—that offer insights into manufacturing automation and innovation.
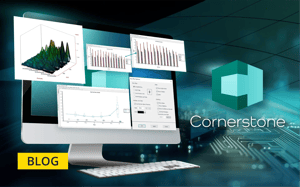
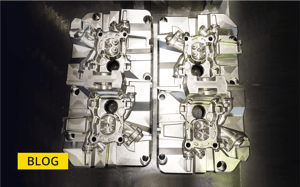
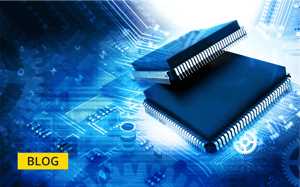
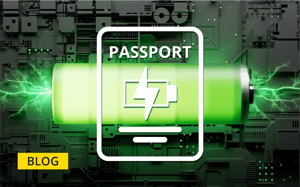
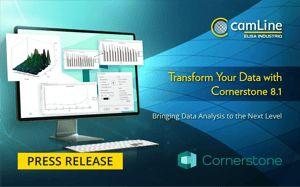


Let’s Discuss Solutions with camLine's Experts
Our team is ready to deliver tailored solutions that streamline your production, improve product quality, and maximize efficiency across your operations. Tap into camLine’s decades of expertise in digital transformation to overcome your manufacturing challenges.